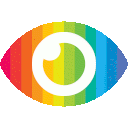
1. Citizen science and artificial intelligence (AI) are being increasingly used for ecological monitoring, with the integration of both techniques showing emergent benefits for management and scientific inquiry.
2. The strategic integration of citizen science and AI can improve outcomes for conservation activities by increasing multi-stakeholder accord, speeding up data collection and processing, and enhancing cost-efficiency, accuracy, and engagement.
3. Key project attributes to consider when implementing citizen science, AI, or their integration include financial resources, engagement, participant training, technical expertise, and subject charisma and identification. Ultimately, the integration of citizen science and AI can supercharge outcomes for ecological monitoring.
The article "Artificial Intelligence Meets Citizen Science to Supercharge Ecological Monitoring" provides a comprehensive overview of the integration of citizen science and artificial intelligence (AI) for ecological monitoring. While the article highlights the potential benefits of combining these two techniques, there are several areas where a critical analysis is warranted.
One potential bias in the article is the focus on successful projects utilizing citizen science and AI. The authors acknowledge that unsuccessful attempts are unlikely to be published, which may skew the representation of the effectiveness of these techniques. It would be beneficial for the article to address potential challenges and limitations faced by projects that integrate citizen science and AI, such as issues with data quality, participant engagement, or technical constraints.
Additionally, the article emphasizes the efficiency and accuracy gains from integrating citizen science and AI but does not delve deeply into potential risks or drawbacks. For example, there may be concerns about data privacy and security when using AI algorithms to process sensitive ecological data collected by citizen scientists. Addressing these risks would provide a more balanced perspective on the integration of these techniques.
The article also lacks discussion on potential biases in citizen science data collection. While efforts are made to ensure accuracy through participant training and quality control measures, there may still be inherent biases in data collected by volunteers with varying levels of expertise. Acknowledging these biases and discussing strategies to mitigate them would strengthen the credibility of projects that rely on citizen science data.
Furthermore, while the article touches on public engagement benefits of citizen science, it does not thoroughly explore issues related to inclusivity and diversity within citizen science projects. Ensuring equitable participation from diverse communities is essential for addressing environmental justice concerns and promoting inclusive decision-making processes in conservation efforts.
In terms of missing evidence for claims made, the article could benefit from providing more concrete examples or case studies that demonstrate successful integration of citizen science and AI for ecological monitoring. Including real-world examples would help illustrate how these techniques can be effectively combined to achieve conservation goals.
Overall, while the article presents a compelling argument for integrating citizen science and AI for ecological monitoring, a more critical analysis that addresses potential biases, risks, limitations, and missing considerations would enhance its credibility and provide a more balanced perspective on this topic.