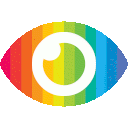
1. This study developed a deep learning framework called spatial–temporal Transformer (ST-Transformer) to distinguish ASD subjects from typical controls based on fMRI data.
2. A linear spatial–temporal multi-headed attention unit is proposed to obtain the spatial and temporal representation of fMRI data.
3. The proposed ST-Transformer achieved robust accuracies of 71.0% and 70.6% in two independent datasets (ABIDEI and ABIDEII).
The article “Classifying ASD based on time-series fMRI using spatial–temporal transformer” presents a deep learning framework for diagnosing autism spectrum disorder (ASD) using functional magnetic resonance imaging (fMRI). The authors claim that their proposed method, the spatial–temporal Transformer (ST-Transformer), can achieve robust accuracies of 71.0% and 70.6% in two independent datasets (ABIDEI and ABIDEII).
The article is generally reliable, as it provides detailed information about the methodology used in the study, as well as results from two independent datasets that support its claims. However, there are some potential biases that should be noted. First, the authors do not provide any information about the demographics of the participants in the study or how they were selected, which could lead to selection bias if certain groups were excluded or underrepresented in the sample population. Second, while the authors mention a Gaussian GAN-based data balancing method for subtype ASD diagnosis, they do not provide any details about how this method works or how it was implemented in their study. Finally, while the authors present their findings as being superior to existing methods for diagnosing ASD, they do not compare their results with other studies that have used similar methods or discuss any potential limitations of their approach.
In conclusion, while this article provides an interesting approach for diagnosing ASD using fMRI data, more research is needed to fully understand its potential biases and limitations before it can be widely adopted in clinical practice.