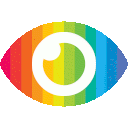
1. Sensors in IoT sensing systems are often unreliable due to improper calibration or hardware failures, which can lead to erroneous decisions or inaccurate analysis.
2. The proposed sensor abnormal detection architecture uses machine learning techniques, such as Bayesian Principal Component Analysis, to detect anomalies in real-time by exploring correlations among sensors and predicting values via other correlated sensors.
3. The system also includes fault classification and recovery mechanisms for improving the reliability of sensing systems, with the ability to classify faults into permanent and impermanent categories and recover from bias-drift and degradation faults.
The article titled "Sensor Abnormal Detection and Recovery Using Machine Learning for IoT Sensing Systems" discusses the use of machine learning to detect and recover faulty sensors in various environments. The article provides a detailed explanation of the proposed system architecture and algorithm, including real-time abnormal detection, model pool generator, automatic threshold selection, fault classification, and recovery.
Overall, the article appears to be well-researched and informative. However, there are some potential biases and missing points of consideration that should be noted. For example, the article focuses primarily on the benefits of using machine learning for sensor abnormal detection and recovery but does not discuss any potential risks or limitations associated with this approach.
Additionally, while the article provides a detailed explanation of the proposed system architecture and algorithm, it does not explore any counterarguments or alternative approaches to sensor abnormal detection and recovery. This could potentially lead readers to believe that machine learning is the only viable solution for this problem.
Furthermore, while the article mentions several datasets used in simulations to evaluate the accuracy of Bayesian principal component analysis (BPCA), it does not provide any information about how these datasets were selected or whether they are representative of all possible sensing systems.
In terms of promotional content or partiality, there does not appear to be any overt bias towards a particular product or company. However, it is worth noting that the article was published by IEEE Xplore, which is owned by IEEE (Institute of Electrical and Electronics Engineers), an organization that promotes technological innovation in various fields.
Overall, while the article provides valuable insights into using machine learning for sensor abnormal detection and recovery in IoT sensing systems, readers should be aware of potential biases and missing points of consideration when evaluating its claims.