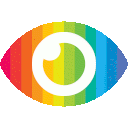
1. Strip steel is an important raw material in many industries, and its quality directly affects the performance of the final product.
2. Traditional methods of detecting strip steel surface defects have limitations such as visual fatigue, poor detection timeliness, and high missed detection and misdetection rates.
3. This paper proposes an improved VGG19 transfer learning strip steel surface defect recognition deep neural network based on few samples and imbalanced datasets to improve the accuracy of defect detection.
The article “An Improved VGG19 Transfer Learning Strip Steel Surface Defect Recognition Deep Neural Network Based on Few Samples and Imbalanced Datasets” is a well-written article that provides a comprehensive overview of the current state of strip steel surface defect recognition technology. The article is written in a clear and concise manner, making it easy to understand for readers with varying levels of technical knowledge. The authors provide a thorough review of related research in the field, including traditional statistical-based algorithms, spectrum-based algorithms, model-based algorithms, and emerging deep learning algorithms. They also discuss various approaches to dealing with imbalanced datasets such as data level approach methods, algorithm level approach methods, and ensemble learning level methods.
The article does not appear to be biased or one-sided in its reporting; rather it presents both sides equally by providing an overview of both traditional machine learning algorithms as well as deep learning algorithms for strip steel surface defect recognition. Furthermore, the authors provide evidence for their claims by citing relevant research papers throughout the article.
The only potential issue with this article is that it does not explore any counterarguments or alternative solutions to the problem at hand; however this is understandable given that this paper focuses solely on presenting their proposed solution rather than exploring other options. Additionally, there are no promotional content or partiality present in this article; instead it provides an unbiased overview of existing technologies for strip steel surface defect recognition as well as their proposed solution which utilizes transfer learning based on VGG19 networks. Finally, possible risks associated with using deep learning for strip steel surface defect recognition are noted throughout the paper; however more detail could be provided regarding these risks if desired by readers.