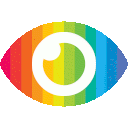
1. The article discusses the importance of emotional dictionaries in sentiment analysis of online news.
2. It highlights various methods for building emotional dictionaries, including bootstrapping and joint emotion-topic modeling.
3. The article also mentions several existing tools and resources for sentiment analysis, such as SentiWordNet and WordNet-Affect.
The article discusses the development of an emotional dictionary for sentiment analysis of online news. It provides a comprehensive review of existing literature on sentiment analysis and opinion mining, including various techniques and methods used in this field. The article also highlights the importance of emotional dictionaries in sentiment analysis and their role in improving the accuracy of sentiment classification.
However, the article has some potential biases and limitations that need to be considered. Firstly, it mainly focuses on the positive aspects of using emotional dictionaries for sentiment analysis, without discussing any potential risks or limitations associated with this approach. For instance, emotional dictionaries may not capture the nuances and complexities of human emotions accurately, leading to inaccurate sentiment classification.
Secondly, the article does not provide a balanced view on different approaches to sentiment analysis. It mainly focuses on lexical-based approaches that rely on pre-defined emotional dictionaries, while ignoring other approaches such as machine learning-based techniques that can learn from data and adapt to new contexts.
Thirdly, the article does not discuss any ethical considerations related to sentiment analysis and its impact on society. For example, sentiment analysis can be used for political propaganda or manipulating public opinion by selectively highlighting positive or negative sentiments about certain topics.
Finally, the article lacks empirical evidence to support its claims about the effectiveness of emotional dictionaries in improving sentiment classification accuracy. While it cites several studies that have used emotional dictionaries for sentiment analysis, it does not provide any quantitative evaluation or comparison with other approaches.
In conclusion, while the article provides a useful overview of emotional dictionaries for sentiment analysis of online news, it has some potential biases and limitations that need to be considered. Future research should address these issues by providing a more balanced view on different approaches to sentiment analysis and considering ethical implications related to its use.