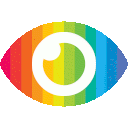
1. The article describes the development of a system that monitors social media for landslide-related content using artificial intelligence to identify and retain relevant information in near-real-time.
2. The system harvests photographs from social media data, tags each image as landslide or not-landslide, and has been validated with a Precision of 76% in detecting landslide reports during real-world deployment.
3. The methodology presented in the article aims to provide a tool for disaster management to automatically detect landslide images from social media, improving response times for first responders and enabling the incorporation of this data into multi-hazard impact assessment workflows.
The article titled "A near-real-time global landslide incident reporting tool demonstrator using social media and artificial intelligence" presents a methodology for monitoring social media for landslide-related content in near-real-time. The article discusses the development of a system that uses artificial intelligence to identify and retain relevant information from social media posts, specifically focusing on images of landslides. The system aims to improve response times for first responders by providing timely data from those affected by landslides.
One potential bias in the article is the focus on Twitter as the primary source of data for landslide reports. While Twitter is a popular platform with a large user base, it may not be representative of all landslide events globally. There are many regions where Twitter usage is limited or non-existent, which could result in underrepresentation of certain areas in the dataset. Additionally, the article does not address potential biases within social media data itself, such as misinformation or false reports that could skew the results.
The article claims that the system can detect landslide reports with a precision of 76%, but it does not provide detailed information on how this metric was calculated or validated. Without transparency on the methodology used to evaluate the system's performance, it is difficult to assess the reliability of this claim.
Furthermore, the article focuses primarily on the benefits of using social media data for landslide monitoring and response, but it does not adequately discuss potential risks or limitations associated with this approach. For example, there may be privacy concerns related to harvesting and analyzing publicly available social media data without explicit consent from users. Additionally, relying solely on social media data may overlook important information from traditional sources such as news outlets or government agencies.
The article also lacks discussion on potential biases introduced during the image labelling process and model training. It is crucial to consider how biases in the training data or annotation process could impact the accuracy and generalizability of the model when applied to real-world scenarios.
Overall, while the article presents an innovative approach to leveraging social media and artificial intelligence for landslide monitoring, there are several gaps and potential biases that need to be addressed for a more comprehensive and balanced analysis. Further research should aim to validate the system's performance using rigorous evaluation methods and consider ethical implications associated with using social media data for disaster response purposes.