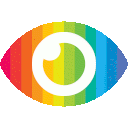
1. Precision medicine for cancer treatment requires understanding the genomic landscape of individual cells and their responses to drugs, which can be highly heterogeneous.
2. Deep transfer learning (DTL) can be used to transfer knowledge and relation patterns from bulk RNA-seq data to single-cell RNA-seq data, enabling the prediction of drug responses at the single-cell level.
3. The scDEAL (single-cell Drug rEsponse AnaLysis) framework uses DTL to predict drug responses in individual cells by harmonizing gene expression features between bulk and single-cell data, incorporating cell cluster labels for regularization, and inferring signature genes of drug response predictions through integrated gradient interpretation.
作为一篇科学论文,该文章提供了一个新的方法来预测单个癌细胞对药物的反应。然而,在阅读文章时,我注意到以下几点:
1. 偏见来源:文章没有提及任何可能存在的风险或限制条件。这种偏见可能会导致读者对该方法的实际应用和效果产生误解。
2. 片面报道:文章只关注了单个癌细胞对药物的反应,但并没有考虑其他因素如患者基因组、环境等可能影响治疗效果的因素。
3. 缺失考虑点:文章没有讨论如何处理数据不平衡问题,即某些药物可能有更多的样本数据可用于训练模型,而其他药物则缺乏足够的数据。
4. 主张缺失证据:文章声称该方法可以预测单个癌细胞对药物的反应,并且在六个基准测试中取得了高精度。然而,作者并未提供足够的证据来支持这一主张。
5. 未探索反驳:文章没有探讨其他可能存在的方法来预测单个癌细胞对药物的反应,并且也没有与现有方法进行比较。
6. 宣传内容:尽管该论文是一篇科学论文,但它似乎试图推销作者开发的新方法,并强调其优势和创新性。
7. 偏袒:文章似乎偏袒使用深度学习技术来解决问题,并未探讨其他可能存在的方法或技术。
总之,尽管该论文提供了一个新颖和有前途的方法来预测单个癌细胞对药物的反应,但它也存在一些偏见和局限性。需要更多研究来验证其有效性和实用性。