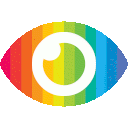
1. The article discusses the use of automated external defibrillators (AEDs) with shock advice algorithms (SAAs) for the rapid diagnosis and treatment of sudden cardiac arrest (SCA).
2. Machine learning (ML) and deep learning (DL) techniques, combined with advanced signal processing methods, have been proposed to improve the performance of SAAs in SCA diagnosis.
3. The article introduces a novel SAA design using principal component analysis (PCA) for feature extraction and a K-nearest neighbor model for classification, resulting in a relatively high performance in SCA detection.
The article titled "Diagnosis of sudden cardiac arrest using principal component analysis in automated external defibrillators" discusses the use of intelligent technologies, specifically machine learning and deep learning, for the diagnosis of sudden cardiac arrest (SCA) using automated external defibrillators (AEDs). The authors highlight the importance of accurate diagnosis in order to provide timely life-support services to SCA patients.
One potential bias in this article is the focus on machine learning and deep learning techniques as the most effective methods for SCA diagnosis. While these techniques have shown promise in previous research, there may be other approaches or combinations of techniques that could also be effective. The article does not explore alternative methods or discuss their potential advantages or disadvantages.
Another potential bias is the emphasis on feature extraction and selection techniques to improve the performance of AEDs. The authors argue that advanced signal processing techniques, such as ensemble empirical mode decomposition and discrete wavelet transform, can enhance the quality of extracted features. However, they do not provide evidence or references to support this claim. Additionally, they do not consider potential limitations or challenges associated with these techniques.
The article also lacks a discussion of potential risks or limitations associated with ML and DL-based SAA designs. For example, ML and DL models require large amounts of data for training, which may be difficult to obtain in certain settings. Additionally, ML models are susceptible to biases present in training data, which could lead to inaccurate diagnoses or unequal treatment outcomes.
Furthermore, the article does not present counterarguments or alternative perspectives on the use of ML and DL for SCA diagnosis. It would be beneficial to include a discussion of potential drawbacks or criticisms of these approaches in order to provide a balanced view.
Overall, while the article provides an overview of current research on SCA diagnosis using intelligent technologies, it has several biases and limitations that should be addressed. A more comprehensive analysis that considers alternative methods, potential risks, and counterarguments would strengthen the article's credibility and provide a more balanced perspective.