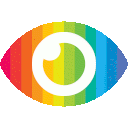
1. Lifelong person re-identification (LRe-ID) is a model that retrieves a person across multiple cameras in continuous data streams and learns incrementally from incoming datasets. However, it faces challenges such as catastrophic forgetting and generalization loss due to parameter updates and domain shifts.
2. The proposed framework, Consistent Region Features Learning (ConRFL), addresses these challenges by sharing consistent features and extracting discriminative features for each sample. It consists of Property Region Features, Feature Adaption, and Feature Perspicacity.
3. The ConRFL framework incorporates a cascade knowledge distillation structure to ensure feature consistency and prevent generalization loss. Experimental results show that the ConRFL framework outperforms state-of-the-art methods in terms of performance improvement.
对于上述文章的详细批判性分析,以下是一些观点和问题:
1. 偏见及其来源:文章没有明确提到作者的背景或利益相关方。这可能导致潜在的偏见,例如作者可能倾向于支持自己的方法而忽视其他方法的优点。
2. 片面报道:文章只关注了作者提出的方法,并没有对其他现有方法进行充分的比较和评估。这可能导致读者对该方法的实际效果和与其他方法相比的优势缺乏全面的了解。
3. 无根据的主张:文章声称该方法在多个Re-ID数据集上取得了显著改进,但没有提供具体的实验证据来支持这一主张。缺乏实验证据使得读者难以评估该方法是否真正有效。
4. 缺失的考虑点:文章没有讨论该方法可能存在的局限性或风险。例如,是否存在过拟合或泛化能力不足等问题?这些因素可能会影响该方法在实际应用中的可行性和效果。
5. 所提出主张的缺失证据:文章声称该方法可以解决LRe-ID中遗忘和泛化损失等挑战,但并未提供充分的证据来支持这些主张。缺乏实验证据使得读者难以相信该方法的有效性。
6. 未探索的反驳:文章没有提及其他学者对于LRe-ID中遗忘和泛化损失问题的不同观点或方法。这可能导致读者对该方法的可行性和优势缺乏全面的了解。
7. 宣传内容:文章中提到将发布源代码,但并未提供任何关于该方法开源程度或可复现性的详细信息。这可能使读者难以评估该方法是否真正具有可操作性。
总体而言,上述文章存在一些潜在的问题和不足之处。为了更好地评估该方法的有效性和实用性,需要更多的实验证据、全面比较和考虑到其他相关研究领域的观点。