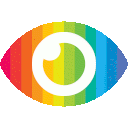
1. Deep learning has achieved great success in image classification, but designing the optimal network structure for different applications is a challenge.
2. Neural Architecture Search (NAS) aims to automatically search for the best deep learning architecture, but initial NAS methods require significant computational resources.
3. Evolutionary Neural Architecture Search (EvoNAS) combines Evolutionary Algorithms with NAS and uses parameter sharing to accelerate the search process. The proposed EvoNAS-Rep algorithm achieves state-of-the-art performance in image classification tasks.
这篇文章介绍了一种基于遗传算法的进化神经架构搜索方法,用于图像分类。然而,文章存在一些潜在的偏见和问题。
首先,文章没有提及其他神经架构搜索方法的优点和缺点。它只关注了Evolutionary Neural Architecture Search (EvoNAS)方法,并未对其他方法进行充分比较和评估。这可能导致读者对该方法的效果和适用性有所误解。
其次,文章没有提供足够的证据来支持作者所提出的主张。虽然文章声称EvoNAS-Rep是一种有效的神经架构搜索方法,但并未提供实验证据或与其他方法进行比较来支持这一观点。缺乏实验证据可能使读者难以相信该方法的可行性和有效性。
此外,文章没有探讨可能存在的风险或局限性。神经架构搜索是一个复杂且计算密集型的任务,可能会面临许多挑战和限制。例如,计算资源需求高、搜索空间巨大等问题都可能影响到该方法的实际应用。
最后,文章没有平等地呈现双方观点。它只关注了EvoNAS-Rep作为一种有效的神经架构搜索方法,并未探讨其他研究人员对该方法的批评或不同观点。这可能导致读者对该方法的评估存在偏见。
综上所述,这篇文章在介绍基于遗传算法的进化神经架构搜索方法时存在一些潜在的偏见和问题。为了提高文章的可信度和客观性,作者应该提供更多实验证据、比较不同方法,并探讨可能存在的风险和局限性。