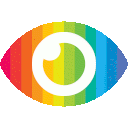
1. Federated Learning (FL) is a collaborative learning approach where local workers train a global model using their local data and communicate trained models to a central server for privacy concerns.
2. FL is subject to various heterogeneities, including system and statistical heterogeneity. To address these concerns, Federated Proximal (FedProx) has been proposed as a promising FL paradigm that provides stable learning convergence in the presence of computation stragglers and statistical heterogeneity.
3. In wireless networks with unreliable communication channels, packet transmission errors introduce additional heterogeneity. This article rigorously proves the convergence of FedProx in the presence of transmission packet errors in heterogeneous networks and proposes a joint client selection and resource allocation strategy to maximize the number of effective participating users for convergence acceleration. The proposed method achieves faster convergence and requires fewer communication rounds compared to existing client selection methods.
对于上述文章的批判性分析,以下是一些可能的观点和问题:
1. 潜在偏见及其来源:文章可能存在潜在的偏见,因为它似乎只关注了Federated Proximal (FedProx)作为解决方案,并没有探讨其他可能的方法或算法。这种偏见可能源自作者对FedProx的研究兴趣或资助机构的要求。
2. 片面报道:文章似乎只关注了FedProx在处理计算滞后和统计异质性方面的优势,而没有提及其他可能存在的问题或挑战。这种片面报道可能导致读者对FL技术整体效果和可行性的误解。
3. 无根据的主张:文章声称提出了一种联合客户端选择和资源分配策略来加速收敛,并且比现有方法更快地达到准确性。然而,文章并未提供足够的证据或实验证明这种策略确实有效。缺乏实验证据使得读者难以评估该方法是否真正具有优势。
4. 缺失的考虑点:文章没有探讨FL在不可靠通信信道中存在的其他潜在问题,如数据传输安全性、隐私保护等。这些是FL领域中重要的考虑因素,但在文章中被忽略了。
5. 所提出主张的缺失证据:文章声称所提出的方法实现了更快的收敛速度和更少的通信轮次,但没有提供充分的证据来支持这些主张。读者需要更多的实验证据来验证这些声明是否成立。
6. 未探索的反驳:文章没有探讨可能存在的反驳观点或对所提出方法的质疑。这种未探索反驳可能导致读者对该方法的可行性和有效性产生疑问。
7. 宣传内容:文章似乎过于宣传所提出方法的优势,而没有充分讨论其局限性或潜在风险。这种宣传内容可能使读者对该方法过于乐观,而忽视了其他可能存在的问题。
8. 偏袒:文章似乎偏向于支持FedProx作为解决方案,并没有平等地呈现其他可能存在的方法或算法。这种偏袒可能影响读者对FL领域整体发展和多样化解决方案的理解。
总之,上述文章在一些方面存在潜在偏见、片面报道、无根据主张、缺失考虑点等问题。读者需要保持批判性思维,并寻找更多的证据和观点来全面评估该文章所提出的主张。