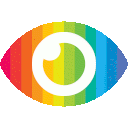
1. The SpatialFormer structure is designed to generate more accurate attention regions based on global features for Few-Shot Learning (FSL).
2. Two specific attention modules, named SpatialFormer Semantic Attention (SFSA) and SpatialFormer Target Attention (SFTA), are derived to enhance the target object regions while reducing background distraction.
3. The proposed method achieves state-of-the-art results on few-shot classification benchmarks.
The article titled "SpatialFormer: Semantic and Target Aware Attentions for Few-Shot Learning" presents a new approach to few-shot learning (FSL) that aims to generate more accurate attention regions based on global features. The authors argue that current CNN-based cross-attention approaches suffer from two problems: inaccurate attention maps based on local features and mutually similar backgrounds causing distraction. To address these issues, the authors propose a novel SpatialFormer structure that explores semantic-level similarity between pair inputs to boost performance.
While the article provides a detailed explanation of the proposed approach, it lacks a critical analysis of potential biases and limitations. For example, the authors do not discuss any potential risks or limitations associated with their approach, such as overfitting or generalization issues. Additionally, the article does not present any counterarguments or alternative approaches to FSL that may be equally effective.
Furthermore, the article contains some promotional content in its claims of achieving new state-of-the-art results on few-shot classification benchmarks without providing sufficient evidence for these claims. The authors also do not acknowledge any potential sources of bias in their research or funding sources.
Overall, while the article presents an interesting approach to FSL, it would benefit from a more critical analysis of potential biases and limitations and a more balanced presentation of alternative approaches and counterarguments.