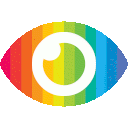
1. A full-resolution convolutional network with a dynamic graph cut algorithm is proposed for skin cancer classification and detection using dermoscopy images.
2. The proposed model solves the common problems of over-segmentation and under-segmentation in the graph cut method, and shows the usefulness of data augmentation in training and testing.
3. Multiple experiments were done using various transferring models, and the results of the recommended model showed superior performance in skin lesion categorization tasks relative to other architectures with an accuracy of 97.986%.
The article titled "A full-resolution convolutional network with a dynamic graph cut algorithm for skin cancer classification and detection" presents a new approach to accurately diagnose skin cancer types using dermoscopy images. The proposed method uses a hyper-parameter optimized full resolution convolutional network with a dynamic graph cut algorithm for accurate segmentation and improved skin cancer classification. The article highlights the importance of early identification of skin cancer and the limitations of current diagnostic methods that rely on visual inspection by dermatologists.
The article provides a comprehensive literature review of previous studies on machine learning methods for skin cancer analysis, highlighting the success of deep learning techniques like Full Resolution Convolutional Networks (FrCN) in automated diagnosis. However, the article also notes the limitations of these approaches, such as overfitting between training and testing phases and the need for more labeling information or complex new training parameters.
The proposed FrCN-DGCA model is presented as an improvement over previous approaches, addressing issues such as over-segmentation and under-segmentation in graph cut methods. The article provides detailed information on the methodology used to optimize hyper-parameters through a dynamic graph cut algorithm method, emphasizing the proper balance between exploration and exploitation.
While the article presents promising results for the proposed model, it does not provide sufficient evidence to support its claims. For example, while it states that multiple experiments were done using various transferring models, it does not provide details on these experiments or how they were conducted. Additionally, there is no discussion of potential risks or limitations associated with this approach.
Furthermore, the article appears to be biased towards promoting the proposed FrCN-DGCA model without exploring counterarguments or presenting both sides equally. While it acknowledges some limitations of previous approaches, it does not provide a balanced perspective on their strengths and weaknesses.
In conclusion, while the proposed FrCN-DGCA model shows promise in accurately diagnosing skin cancer types using dermoscopy images, further research is needed to validate its effectiveness and address potential limitations. The article could benefit from a more balanced perspective and a more detailed discussion of the methodology used in experiments.