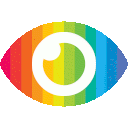
1. The problem of retrieving a specific moment from an untrimmed video by a query sentence is challenging due to temporal dependencies.
2. A two-dimensional map is proposed to model the temporal relations between video moments, allowing for diverse moments with different lengths to be represented.
3. The proposed Temporal Adjacent Network (2D-TAN) outperforms state-of-the-art methods on three challenging benchmarks.
The article proposes a novel approach to the problem of retrieving a specific moment from an untrimmed video by a query sentence. The proposed method, called Temporal Adjacent Network (2D-TAN), models the temporal relations between video moments by a two-dimensional map and is capable of encoding adjacent temporal relations while learning discriminative features for matching video moments with referring expressions.
The article provides a clear and concise explanation of the proposed method and its advantages over existing methods. The authors also provide experimental results on three challenging benchmarks, i.e., Charades-STA, ActivityNet Captions, and TACoS, where their 2D-TAN outperforms the state-of-the-art.
However, there are some potential biases in the article that need to be considered. Firstly, the authors do not mention any limitations or potential risks associated with their proposed method. It is important to note that any new technology or method may have unintended consequences or limitations that need to be addressed.
Secondly, the authors do not explore any counterarguments or alternative approaches to solving the problem of moment localization with natural language. This may give readers the impression that their proposed method is the only viable solution to this problem.
Thirdly, the article does not provide enough evidence for some of its claims. For example, it claims that existing methods cannot tackle this challenge well since they consider temporal moments individually and neglect temporal dependencies. However, no evidence is provided to support this claim.
Finally, there is some promotional content in the article as it emphasizes how their proposed method outperforms state-of-the-art methods on three challenging benchmarks. While this is certainly impressive, it may give readers an overly positive view of their proposed method without considering its limitations or potential risks.
In conclusion, while the article presents an interesting and potentially useful approach to moment localization with natural language, readers should be aware of its potential biases and limitations. It would be beneficial for future research in this area to explore alternative approaches and consider potential risks associated with new methods.