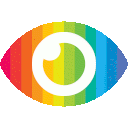
1. A surrogate modeling method based on deep learning has been developed for distributed land surface hydrological models.
2. The surrogate model can handle high-dimensional input-output maps and capture the dynamic relationship between the time-varying input and output.
3. The deep autoregressive neural network used in the surrogate model outperforms the LSTM model in approximating spatial patterns of VIC simulated soil moisture.
The article titled "A surrogate modeling method for distributed land surface hydrological models based on deep learning" presents a new approach to developing surrogate models for distributed hydrological models using deep learning techniques. The authors claim that their method can handle high-dimensional input-output maps and capture the dynamic relationship between the time-varying input and output. They also state that their surrogate model outperforms the popular LSTM model in approximating spatial patterns of VIC output.
Overall, the article provides a detailed description of the methodology used and presents results that support the authors' claims. However, there are several points that need to be critically analyzed.
Firstly, the article does not provide a comprehensive discussion of the limitations and potential biases of the proposed method. While the authors mention that traditional surrogate techniques have trouble in modeling high-dimensional input-output maps, they do not discuss why this is the case or how their method overcomes these challenges. Additionally, there is no discussion of potential sources of bias in the data used to train and test the surrogate model.
Secondly, the article does not provide sufficient evidence to support some of its claims. For example, while the authors state that their surrogate model significantly outperforms the LSTM model in approximating spatial patterns of VIC output, they do not provide any statistical analysis or comparison between the two models. Without such evidence, it is difficult to determine whether these claims are valid.
Furthermore, there are missing points of consideration in the article. For instance, there is no discussion of potential risks or limitations associated with using deep learning techniques for developing surrogate models. Deep learning models are known to be computationally expensive and require large amounts of training data. The article does not address how these challenges were overcome or whether they could limit the applicability of their method in real-world scenarios.
Additionally, there is a lack of exploration of counterarguments or alternative approaches to developing surrogate models for distributed hydrological models. The article focuses solely on the proposed deep learning method and does not discuss other existing methods or their potential advantages or disadvantages.
Finally, the article has a promotional tone, with the authors emphasizing the superiority of their method over existing approaches. This raises concerns about potential bias and conflicts of interest. It would have been more balanced if the authors had provided a more objective assessment of their method and compared it to other approaches in a fair manner.
In conclusion, while the article presents an interesting approach to developing surrogate models for distributed hydrological models, there are several limitations and biases that need to be critically analyzed. The lack of discussion on potential limitations, missing evidence for claims made, unexplored counterarguments, and promotional tone raise concerns about the validity and objectivity of the findings. Further research and analysis are needed to fully evaluate the proposed method and its applicability in real-world scenarios.