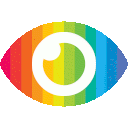
1. Biopharma research in drug R&D remains expensive and time-consuming, but AI can help identify the most promising compounds and targets at every step of the value chain so that fewer, more successful experiments are conducted in the lab to achieve the same number of leads.
2. The AI-driven drug discovery industry has grown significantly over the past decade, with nearly 270 companies working in this space. However, partnerships between traditional biopharma companies and AI-driven drug companies often face obstacles such as keeping AI-enabled discovery approaches at arm's length from internal R&D and investment in digitized drug discovery capabilities and data sets within internal R&D teams being used to leverage partner platforms rather than building end-to-end tech stack and capabilities.
3. Biopharma companies that maximize the impact of AI enablement can move beyond minimum viable product (MVP) individual use cases and build research systems that harness synergies created by putting AI at the center of the research engine to enhance the outcome of experiments. These research systems reduce overall costs, have higher probability of success, accelerate R&D processes (and therefore time to patient impact), and are fully integrated for specific use cases.
The article "AI in biopharma research: A time to focus and scale" by McKinsey provides an overview of the current state of AI-driven drug discovery in the biopharma industry. The article highlights the potential benefits of AI in drug R&D, including enhanced productivity and probability-of-success gains. However, it also acknowledges that there are obstacles to unlocking the full potential of AI enablement, such as keeping AI-enabled discovery approaches at arm's length from internal R&D and investing in digitized drug discovery capabilities and data sets within internal R&D teams.
One potential bias in the article is its focus on the benefits of AI-driven drug discovery without fully exploring its potential risks. For example, there is a risk that relying too heavily on AI could lead to overreliance on certain algorithms or models, which could result in biased or inaccurate results. Additionally, there is a risk that AI-driven drug discovery could lead to a reduction in human involvement in the research process, which could have ethical implications.
Another potential bias is the article's emphasis on partnerships between traditional biopharma companies and AI-driven drug companies as a way to maximize the impact of AI enablement. While partnerships can be beneficial, they may not always be necessary or appropriate for every company. Some companies may prefer to build their own internal capabilities rather than partnering with external providers.
The article also makes some unsupported claims, such as stating that "AI can help identify the most promising compounds and targets at every step of the value chain so that fewer, more successful experiments are conducted." While this may be true in some cases, it is not necessarily true for all compounds or targets.
Overall, while the article provides useful insights into the potential benefits and challenges of AI-driven drug discovery in biopharma research, it would benefit from a more balanced discussion of both its benefits and risks. Additionally, it could provide more evidence for some of its claims and explore counterarguments to its points.