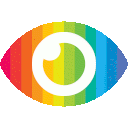
1. Robotic Assisted Minimally Invasive Surgery (RAMIS) lacks force feedback, which can result in tissue damage and malfunctions.
2. Sensorless force estimation is a potential solution for RAMIS, with vision-based force sensing (VBFS) being a promising approach.
3. Deep neural networks, specifically CNNs and LSTM networks, can be effective models for processing video sequences in VBFS for force estimation in robotic surgery.
The article titled "A recurrent convolutional neural network approach for sensorless force estimation in robotic surgery" discusses the challenges of providing force feedback in Robotic Assisted Minimally Invasive Surgery (RAMIS) and proposes a solution using Vision-Based Force Sensing (VBFS) with deep neural networks. While the article provides valuable insights into the potential benefits of VBFS, it also has some limitations and biases that need to be considered.
One-sided reporting: The article focuses primarily on the benefits of VBFS and does not provide a balanced view of its limitations. For example, it does not discuss the potential risks associated with relying solely on video data for force estimation, such as inaccuracies due to camera movement or occlusion.
Unsupported claims: The article makes several claims about the potential benefits of force feedback in RAMIS without providing evidence to support them. For instance, it states that force feedback can result in less intraoperative tissue damage produced by excessive forces, but there is no reference to any studies or research that supports this claim.
Missing points of consideration: The article does not address some critical points related to VBFS, such as the impact of lighting conditions on video quality or the need for accurate calibration of cameras and instruments. These factors can significantly affect the accuracy of force estimates obtained through VBFS.
Missing evidence for claims made: The article suggests that VBFS can restore force feedback in robotic surgery without providing sufficient evidence to support this claim. While some studies have shown promising results with VBFS, more research is needed to validate its effectiveness in clinical settings.
Unexplored counterarguments: The article does not explore alternative approaches to sensorless force estimation, such as control-based methods that use models and observers to estimate interaction forces. This omission limits the reader's understanding of the relative strengths and weaknesses of different approaches.
Promotional content: The article appears to promote the proposed approach using deep neural networks without acknowledging its limitations or potential drawbacks. This bias may be due to the authors' affiliation with institutions that specialize in artificial intelligence and robotics research.
Partiality: The article focuses exclusively on VBFS using deep neural networks and does not consider other types of machine learning algorithms or techniques for processing video data. This partiality limits the scope of discussion and may lead readers to believe that deep learning is the only viable approach for sensorless force estimation.
In conclusion, while the proposed approach using deep neural networks for sensorless force estimation shows promise, there are limitations and biases in this article that need to be considered. A more balanced discussion would provide a clearer understanding of both the potential benefits and limitations associated with VBFS using deep learning algorithms.