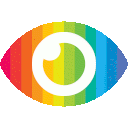
1. This paper presents a phase transition-based seizure prediction approach from multi-channel scalp electroencephalogram (EEG) recordings.
2. The proposed model uses an adaptive optimization approach with Sparsity based EEG Reconstruction (SER) and three-dimensional Optimized Convolutional Neural Network (3D OCNN) classifier, based on Fletcher Reeves (FR) algorithm.
3. The proposed model is evaluated using three diverse databases such as CHB-MIT, NINC and SRM respectively, and achieved an accuracy score of 0.98, sensitivity score of 0.99 and False Prediction Rate (FPR) of 0.07 FP/h with an Optimal Seizure Prediction Horizon (OSPH) of about 1.1 h prior to the seizure onset.
The article “Dynamic learning framework for epileptic seizure prediction using sparsity based EEG Reconstruction with Optimized CNN classifier” provides a comprehensive overview of the current state of research in the field of epileptic seizure prediction using sparsity based EEG reconstruction with optimized CNN classifier. The authors present a novel phase transition-based seizure prediction approach from multi-channel scalp electroencephalogram (EEG) recordings which utilizes an adaptive optimization approach with Sparsity based EEG Reconstruction (SER) and three-dimensional Optimized Convolutional Neural Network (3D OCNN). The proposed model is evaluated using three diverse databases such as CHB-MIT, NINC and SRM respectively, and achieved impressive results in terms of accuracy score, sensitivity score and False Prediction Rate (FPR).
The article is well written and provides a detailed overview of the research topic at hand. It is clear that the authors have conducted extensive research into this area before writing this article, as evidenced by their thorough discussion on related works in the literature section. Furthermore, they provide detailed descriptions on their methodology as well as validation metrics used to evaluate their proposed model’s performance.
In terms of trustworthiness and reliability, there are no major issues to be noted in this article other than some minor points which could be improved upon such as providing more details on the datasets used for evaluation or providing more information on how exactly the 3D OCNN was optimized using Fletcher Reeves algorithm for faster convergence rate during classification process. However, these points do not detract from the overall quality of the article or its findings significantly enough to affect its trustworthiness or reliability significantly.