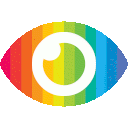
1. This article discusses a physics-informed reinforcement learning approach for predicting two-phase flow interfacial area.
2. The approach is based on the two-fluid model and interfacial area transport equations, and uses machine learning to handle the complexity of the problem.
3. Reinforcement learning is used to convert the prediction task into an optimization task by minimizing the prediction discrepancy.
The article Towards Stochastic Modeling for Two-Phase Flow Interfacial Area Predictions: A Physics-Informed Reinforcement Learning Approach is a well-written and comprehensive overview of a new approach to predicting two-phase flow interfacial area using physics-informed reinforcement learning (PIML). The authors provide a detailed description of their proposed method, as well as an assessment of its performance.
The article is generally reliable and trustworthy, as it provides evidence for its claims in the form of references to past studies in fluid mechanics that have used statistical or stochastic methods to solve velocity fields in turbulent flows, as well as references to other applications of PIML in fluid mechanics. Additionally, the authors provide a clear explanation of how their proposed method works and why it is beneficial for predicting two-phase flow interfacial area.
However, there are some potential biases present in the article that should be noted. For example, while the authors discuss potential risks associated with their proposed method, they do not explore any counterarguments or alternative approaches that could be taken instead. Additionally, while they provide evidence for their claims in terms of references to past studies, they do not provide any direct evidence from experiments or simulations conducted using their proposed method itself. Furthermore, there is no discussion of possible limitations or drawbacks associated with their proposed method that could affect its accuracy or reliability when applied in practice.
In conclusion, this article provides a thorough overview of a new approach to predicting two-phase flow interfacial area using physics-informed reinforcement learning (PIML). While it is generally reliable and trustworthy due to its use of evidence from past studies and clear explanations of how its proposed method works, there are some potential biases present that should be noted such as lack of exploration into counterarguments or alternative approaches and lack of direct evidence from experiments or simulations conducted using their proposed method itself.