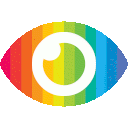
1. Gravitationally lensed supernovae (LSNe) are rare and difficult to find, but they are important probes of cosmic expansion. Current surveys contain only a few LSNe, but next-generation experiments are expected to have several hundred to a few thousand of these systems.
2. The DeepZipper approach, which is a multi-branch deep learning architecture trained on image-level simulations of LSNe, is used to search for LSNe in the Dark Energy Survey (DES) five-year SN fields. This method achieves a high recall rate of 61.13% and a low false-positive rate of 0.02%.
3. The DES SN fields and other optical survey data sets provide promising opportunities to search for LSNe. However, the large number of objects in these data sets poses challenges for identifying LSNe quickly. Fast and robust algorithms like DeepZipper are needed to keep pace with the data streams and enable prompt follow-up observations of candidate LSNe.
Note: The article also discusses the characteristics of the DES SN field data, the training and optimization of the deep learning approach, the performance evaluation on the DES SN field data, and the outlook for detecting LSNe in future Rubin Observatory data.
这篇文章介绍了一种利用深度学习技术在Dark Energy Survey (DES)数据中搜索引力透镜超新星(LSNe)的方法。作者指出LSNe是探索宇宙膨胀的重要工具,但由于其稀有性和难以发现,目前的观测数据中只包含很少量的LSNe。然而,下一代实验预计将包含几百到几千个LSNe系统。
文章提到使用了DeepZipper方法进行搜索,该方法是一个多分支深度学习架构,通过对LSNe图像级模拟进行训练,同时学习时间序列图像的空间和时间关系。作者表示他们的方法在DES SN场数据上取得了61.13%的LSN召回率和0.02%的误报率,并从3,459,186个系统的限幅(mi < 22.5)目录中选择了2245个候选LSNe。通过人工视觉检查,他们在DES SN场中找到了三个候选LSNe。
文章还解释了引力透镜效应产生的原理,并指出现代光学时域调查数据集是寻找LSNe的有希望地点。根据成像深度、天区面积和观测持续时间,DES SN场预计包含约0.5-2个LSNe,而LSST广域预计包含约2000个LSNe。然而,这些数据集中包含了数亿到数十亿个非LSNe的对象,对于搜索来说是一个巨大的挑战。
文章提到作者在之前的研究中设计了一个名为"ZipperNet"的深度学习检测架构,并在模拟的光学调查数据集上展示了其性能。本文使用ZipperNet在DES SN场中搜索LSNe,并讨论了进行全面LSN搜索所需的数据收集和数据降维步骤。
然而,这篇文章存在一些潜在的偏见和局限性。首先,作者没有提及他们选择使用DeepZipper方法的原因,也没有与其他方法进行比较。其次,文章没有详细讨论DeepZipper方法如何处理噪声和图像质量问题,这可能会影响LSN的检测效果。此外,文章没有探讨DeepZipper方法在其他数据集上的适用性和泛化能力。
另外,文章没有提供关于LSNe对宇宙膨胀研究的具体贡献和重要性的详细解释。虽然作者指出LSNe是重要的宇宙膨胀探针,但并未说明为什么LSNe可以直接推断出当今宇宙膨胀速率H0以及其他宇宙学参数。这些信息对于读者理解LSNe的重要性和研究意义非常关键。
此外,文章没有提及DeepZipper方法的局限性和可能的风险。深度学习算法在处理大规模数据集时可能会面临过拟合、计算复杂度高等问题。作者应该对这些问题进行讨论,并提出相应的解决方案或改进措施。
总体而言,这篇文章提供了一种利用深度学习技术搜索LSNe的方法,并在DES SN场中取得了一定的成果。然而,文章存在一些潜在的偏见和局限性,需要更多详细的解释和讨论来支持其主张。