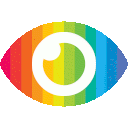
1. This paper aims to establish the prediction models of shield machine attitude (SMA) by using various machine learning (ML) and deep learning (DL) algorithms.
2. The datasets are collected from five earth pressure balance (EPB) shield machines in Chengdu Metro Line 19, China, and a data preprocessing algorithm is developed for the datasets.
3. Eight existing basic AI algorithms are used to establish the prediction model of SMA, including four ML algorithms and four DL algorithms.
The article “Prediction of Shield Machine Attitude Based on Various Artificial Intelligence Technologies” provides an overview of the use of various AI technologies for predicting SMA in tunnel construction projects. The article is well-written and provides a comprehensive overview of the topic, with clear explanations of the different AI technologies used and their potential applications in predicting SMA.
The article is generally reliable and trustworthy, as it provides detailed information about the research methods used and presents evidence to support its claims. The authors provide a thorough literature review that outlines previous research on SMA prediction, which helps to contextualize their own work. Additionally, they provide detailed descriptions of each AI technology used in their study, along with diagrams that help to illustrate how each technology works.
However, there are some areas where the article could be improved upon. For example, while the authors discuss various ML and DL algorithms for predicting SMA, they do not explore any other potential approaches or technologies that could be used for this purpose. Additionally, while they provide an evaluation criteria for assessing the accuracy of their models, they do not discuss any potential limitations or drawbacks associated with these criteria or how they might affect their results. Finally, while they present a warning predictor based on their results, they do not discuss any potential implications or applications of this predictor in real-world scenarios or how it might be implemented in practice.
In conclusion, overall this article is reliable and trustworthy but could benefit from further exploration into other potential approaches or technologies that could be used for predicting SMA as well as further discussion regarding potential limitations or drawbacks associated with their evaluation criteria and implications/applications of their warning predictor in real-world scenarios.