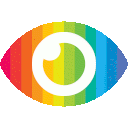
1. Large language models (LLMs) often compare their performance to "human" standards, but fail to consider the cultural diversity and psychological differences among humans globally.
2. LLMs' responses to psychological measures align closely with individuals from Western, Educated, Industrialized, Rich, and Democratic (WEIRD) societies, showing a decline in performance as we move away from these populations.
3. Ignoring cross-cultural diversity in both human and machine psychology can lead to scientific and ethical issues, highlighting the need to address WEIRD bias in future generations of generative language models.
The article "Which Humans?" raises important questions about the potential biases in large language models (LLMs) and their comparison to human performance. The authors highlight the fact that humans are a culturally diverse species, and this diversity is not fully captured in the training data of current LLMs. They argue that LLMs' responses to psychological measures align more closely with individuals from Western, Educated, Industrialized, Rich, and Democratic (WEIRD) societies, leading to a bias in their performance.
One potential bias in the article is the focus on Western societies as the standard for comparison. While it is true that much of the existing research on psychology and cognitive tasks has been conducted in WEIRD populations, it is important to consider that there are other cultural groups with different perspectives and ways of thinking. By solely focusing on WEIRD societies, the authors may overlook valuable insights from non-WEIRD populations.
Additionally, the article makes claims about the decline in LLMs' performance as we move away from WEIRD populations without providing sufficient evidence to support this assertion. It would be beneficial for the authors to include specific examples or studies that demonstrate this decline in performance across different cultural groups.
Furthermore, while the article discusses potential biases in LLMs and calls for mitigating these biases in future generations of generative language models, it does not explore potential solutions or strategies for addressing these issues. Providing concrete recommendations for how researchers can incorporate cross-cultural diversity into LLM training data would strengthen the argument presented in the article.
Overall, while "Which Humans?" raises important considerations about cultural diversity and bias in LLMs, there are areas where further exploration and evidence could enhance the validity of its claims. By acknowledging alternative perspectives, providing more robust evidence for assertions, and offering practical solutions for addressing biases, the article could contribute more effectively to discussions on mitigating bias in generative language models.