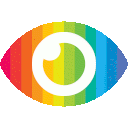
1. Deep convolutional neural networks (CNNs) are widely used and efficient in medical image processing, as they can automatically extract features and represent images optimally.
2. CNN architectures have been extensively studied and applied in various medical imaging modalities for analysis and diagnosis.
3. The use of deep learning techniques, such as CNNs, in medical image processing provides significant advantages, including improved accuracy, reduced human intervention, and faster diagnosis of diseases.
The article titled "Deep convolutional neural network in medical image processing" provides an overview of the use of deep learning techniques, specifically convolutional neural networks (CNNs), in medical image processing. The authors discuss different CNN architectures and their applications in the medical imaging domain, as well as provide a comparison of existing works based on CNNs. They also highlight future challenges and potential solutions.
Overall, the article provides a comprehensive overview of the topic and presents relevant information about the use of CNNs in medical image processing. However, there are some potential biases and limitations that should be considered.
Firstly, the article focuses primarily on the benefits and advantages of using CNNs in medical image processing. While it acknowledges that traditional machine learning techniques require hand-crafted features, it does not provide a balanced discussion of the limitations or drawbacks of CNNs. For example, it does not mention potential issues such as overfitting, interpretability of results, or the need for large amounts of labeled data for training.
Additionally, the article does not provide sufficient evidence or references to support some of its claims. For example, it states that deep learning techniques automatically extract features and use them for diagnosis without requiring hand-crafted features. However, no specific studies or research papers are cited to support this claim.
Furthermore, there is a lack of discussion on potential risks or ethical considerations associated with using deep learning techniques in medical image processing. For example, there could be concerns about privacy and security when dealing with sensitive patient data or potential biases in algorithms that could lead to disparities in healthcare outcomes.
The article also lacks a critical analysis of alternative approaches or methods for medical image processing. While it briefly mentions supervised learning as a popular technique before the advent of deep learning, it does not explore other possible approaches or compare their effectiveness with CNNs.
In terms of reporting bias, the article seems to focus more on promoting the use of CNNs rather than providing a balanced view of the topic. It does not adequately address potential limitations or challenges associated with CNNs and does not present alternative viewpoints or counterarguments.
In conclusion, while the article provides valuable information about the use of CNNs in medical image processing, it has some limitations and biases that should be taken into consideration. A more balanced and critical analysis of the topic, including potential drawbacks and alternative approaches, would enhance the overall quality and credibility of the article.