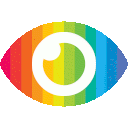
1. PQDs (power quality disturbances) are deviations from the ideal waveform in a power system and can cause operational problems and damage to equipment.
2. Traditional approaches to PQD classification involve feature extraction and classification algorithms, but selecting hand-crafted features requires substantial effort and unbalanced training sets may not be suitable for supervised learning methods.
3. Deep learning (DL) has been proposed as an alternative approach to PQD classification, which involves learning high-level features from data in a hierarchical manner using stacked layer-wise architectures. DL has advantages over traditional methods in terms of accuracy and efficiency.
该文章主要介绍了电力质量扰动的分类方法,以及自动分类方法的发展趋势。然而,该文章存在一些潜在偏见和不足之处。
首先,该文章没有充分考虑到数据采集和处理过程中可能存在的误差和不确定性。例如,由于测量设备的限制或环境噪声等因素,实际采集到的数据可能会受到干扰或失真。此外,在进行特征提取和分类算法时,也需要考虑到模型选择、参数调整等问题。
其次,该文章没有探讨深度学习方法在电力质量扰动分类中的局限性。尽管深度学习具有强大的表达能力和泛化能力,但其对于小样本、非平稳信号等情况下的适应性仍然存在挑战。因此,在实际应用中需要谨慎评估其效果并结合其他方法进行优化。
此外,该文章未涉及到电力质量扰动对人体健康和环境影响等方面的讨论。事实上,一些PQDs如谐波、闪变等已被证明会对人体产生不良影响,并引起社会关注。因此,在研究电力质量扰动分类方法时,也需要考虑到其实际应用和社会责任。
综上所述,该文章虽然介绍了电力质量扰动分类的一些方法和趋势,但在数据处理、深度学习方法的局限性、社会影响等方面存在不足之处。因此,在进行相关研究时需要更加全面地考虑各种因素,并结合实际情况进行优化。