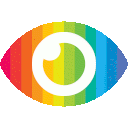
1. Algorithms are increasingly making decisions that were previously made by humans in various fields, such as healthcare, transportation, and law enforcement.
2. People's perceptions of algorithmic decisions may be influenced by their knowledge of the decision-maker being an algorithm or a human.
3. Perceptions of fairness, trust, and emotional responses play a crucial role in people's attitudes towards algorithmic decision-making and can impact collaboration and job satisfaction.
The article "Understanding perception of algorithmic decisions: Fairness, trust, and emotion in response to algorithmic management" by Min Kyung Lee explores how people perceive algorithmic decision-making in managerial contexts. The author argues that the increasing use of algorithms for managerial decisions may influence people's perceptions of the fairness and trustworthiness of those decisions, regardless of their actual outcomes. The article also highlights the importance of understanding societal attitudes towards this change and building a knowledge base to advance people's theories and mental models of algorithmic technology.
The article provides a comprehensive overview of the current state of research on people's perceptions of algorithms, highlighting previous studies that have investigated how people interact with computers as if they were social agents. However, the author acknowledges that the context investigated in this study is different from previous studies since most previous studies used computers and automation technologies as decision aids or interactive partners. This study focuses on how people perceive algorithmic decision-making in managerial contexts where algorithms are increasingly making more decisions that human managers used to make.
One potential bias in this article is its focus on only two factors - fairness and trust - when examining people's perceptions of algorithmic decision-making. While these factors are important, there may be other factors that influence people's perceptions, such as transparency or accountability. Additionally, the article does not explore potential counterarguments to its claims about the influence of algorithmic decision-making on perceptions of fairness and trust.
Another potential bias is the lack of consideration given to possible risks associated with increased reliance on algorithms for managerial decisions. For example, there may be unintended consequences or biases in algorithmic decisions that could negatively impact certain groups or individuals. The article does not address these potential risks or suggest ways to mitigate them.
Overall, while this article provides valuable insights into how people perceive algorithmic decision-making in managerial contexts, it could benefit from a more balanced approach that considers potential biases and risks associated with increased reliance on algorithms.