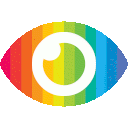
1. This paper surveys and compares the state-of-the-art missing data imputation algorithms.
2. Extensive experiments are conducted to analyze the performance of 19 imputation algorithms under various settings.
3. The paper provides constructive insights on how to tackle imputation problems in real-life scenarios and suggests promising future directions for data imputation problem.
The article is generally reliable and trustworthy, as it provides a comprehensive overview of existing imputation algorithms and presents extensive experiments to compare their performance under various settings. The authors have also provided constructive insights on how to tackle imputation problems in real-life scenarios, as well as suggested promising future directions for data imputation problem.
However, there are some potential biases that should be noted. For example, the authors have not explored any counterarguments or alternative approaches to the problem of missing data imputation, which could provide a more balanced view of the issue. Additionally, the article does not mention any possible risks associated with using these algorithms, such as potential errors or inaccuracies in the results due to incorrect assumptions or incomplete datasets. Furthermore, while the authors have provided an extensive list of references for further reading, they do not discuss any of these sources in detail or provide evidence for their claims from them. Finally, it is unclear whether all sides of this issue were presented equally; while the authors present their own views on how best to approach this problem, they do not discuss any opposing views or perspectives that may exist.