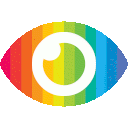
1. Graph-based semi-supervised learning is a hot topic in machine learning and pattern recognition, and constructing an informative graph is crucial for the final performance of learning algorithms.
2. The proposed graph construction method, SRLS, is based on data self-representativeness and Laplacian smoothness, and incorporates an adaptive coding scheme to obtain a sparse graph. Two kernelized versions of SRLS are also introduced.
3. Experiments on several public image datasets show that the proposed methods outperform many state-of-the-art methods, demonstrating the power of Laplacian smoothness as a tool for obtaining informative graphs.
该文章介绍了一种基于数据自我表征和拉普拉斯平滑性的图构建方法,并提出了两个核化版本。作者声称该方法在多个公共图像数据集上的实验表明,该方法可以优于许多最先进的方法。然而,该文章存在以下问题:
1. 偏见来源:文章没有提及其他可能存在的图构建方法,也没有对比分析不同方法之间的优缺点。这可能导致读者对该方法的评估存在偏见。
2. 片面报道:文章只介绍了该方法的优点,但没有详细说明其局限性和适用范围。这可能会误导读者对该方法的理解。
3. 无根据主张:文章声称Laplacian smoothness criterion是一个强大的工具来获取信息图形,但没有提供足够的证据来支持这一主张。
4. 缺失考虑点:文章没有考虑到参数设置对图构建结果影响较大这一问题,并且没有提供如何选择最佳参数设置的解决方案。
5. 主张缺失证据:文章声称SRLS可以同时满足数据自我表征和拉普拉斯平滑性这两个标准,但并未提供充分证据来支持这一主张。
6. 未探索反驳:文章没有探讨其他学者对该方法的反驳和批评,这可能会导致读者对该方法的评估存在偏见。
7. 宣传内容:文章过于强调该方法的优点,而忽略了其局限性和适用范围。这可能会误导读者对该方法的理解。
8. 偏袒:文章没有平等地呈现不同方法之间的优缺点,而是只介绍了该方法的优点。这可能会导致读者对该方法的评估存在偏见。
9. 未注意到可能的风险:文章没有探讨使用该方法可能带来的风险和潜在问题,这可能会误导读者对该方法的应用。
总之,虽然该文章提出了一种新颖的图构建方法,并在实验中取得了良好效果,但其存在上述问题需要进一步完善和改进。