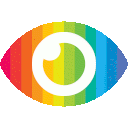
1. A method has been developed to predict daily activities of an individual using images taken from a passive egocentric wearable camera and contextual information such as time and day of the week.
2. The method uses a Convolutional Neural Network (CNN) with a classification method called a late fusion ensemble, which incorporates relevant contextual information and increases classification accuracy.
3. The technique achieves an overall accuracy of 83.07% in predicting a person’s activity across 19 activity classes, and can be fine-tuned for other users with minimal data and annotation.
The article "Predicting Daily Activities From Egocentric Images Using Deep Learning" presents a method for analyzing images taken from a wearable camera to predict daily activities of an individual. The authors collected a dataset of 40,103 egocentric images over six months with 19 activity classes and used deep learning techniques to achieve an overall accuracy of 83.07% in predicting a person's activity.
The article provides a comprehensive review of related work on activity analysis and privacy concerns related to wearable cameras. However, the authors do not address potential biases in their dataset or methodology. For example, the dataset was collected from only one individual, which may limit the generalizability of the results. Additionally, the authors do not discuss potential biases in the annotation process or how they addressed inter-rater reliability.
The article also lacks discussion on potential risks associated with using wearable cameras for continuous and automatic capture of first-person point-of-view images. While the authors briefly mention privacy concerns, they do not provide detailed information on how they addressed these concerns during data collection or analysis.
Furthermore, the article does not explore counterarguments or limitations of their approach. For instance, while deep learning techniques have shown promise in image classification tasks, they are often criticized for being black-box models that lack interpretability. The authors could have discussed potential limitations of their approach and alternative methods for predicting daily activities.
Overall, while the article presents an interesting method for predicting daily activities from egocentric images using deep learning techniques, it lacks critical analysis and discussion on potential biases and limitations of their approach.