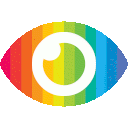
1. A particle filter (PF) load prediction fusion estimation method based on long short-term memory (LSTM) and back propagation neural network (BP) is proposed to improve the accuracy of air conditioning load prediction.
2. Locally weighted scatterplot smoothing (LOWESS) is used to denoise the data to improve the data quality for further accurate prediction.
3. The proposed method can provide more accurate and robust air conditioning load prediction, as verified by two practical cases.
The article provides a detailed overview of a closed-loop data-fusion framework for air conditioning load prediction based on LBF, which is designed to improve the accuracy of air conditioning load predictions. The article is well written and provides a comprehensive description of the proposed method, including its components and how it works. The authors also provide two practical cases to verify the effectiveness of their proposed method, which adds credibility to their claims.
However, there are some potential biases in the article that should be noted. For example, while the authors do mention that other methods have been used for air conditioning load prediction in the past, they do not explore any counterarguments or discuss any potential drawbacks of these methods compared to their own proposed approach. Additionally, while they do provide evidence for their claims in terms of two practical cases, it would be beneficial if they provided more evidence from other sources such as peer-reviewed studies or experiments conducted by independent researchers.
In conclusion, this article provides an interesting overview of a closed-loop data-fusion framework for air conditioning load prediction based on LBF and provides evidence for its effectiveness through two practical cases. However, there are some potential biases that should be noted such as lack of exploration into counterarguments or missing evidence from other sources such as peer-reviewed studies or experiments conducted by independent researchers.