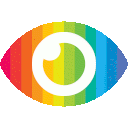
1. The article discusses the use of machine learning in empirical asset pricing, which involves using algorithms to analyze large amounts of financial data and make predictions about future market trends.
2. The authors argue that machine learning can be a valuable tool for investors and financial analysts, as it allows them to quickly process vast amounts of information and identify patterns that might not be visible to the human eye.
3. However, the article also notes that there are potential drawbacks to relying too heavily on machine learning in finance, such as the risk of overfitting models or failing to account for unexpected events that could impact market behavior.
The article titled "Empirical Asset Pricing via Machine Learning" discusses the use of machine learning in empirical asset pricing. The authors, Gu, Kelly, and Xiu, argue that machine learning can improve the accuracy of asset pricing models by incorporating more information and capturing nonlinear relationships between variables.
The article provides a detailed overview of the different types of machine learning algorithms used in empirical asset pricing, including decision trees, random forests, neural networks, and support vector machines. The authors also discuss the challenges associated with using machine learning in finance, such as overfitting and data snooping bias.
Overall, the article is well-written and informative. However, there are some potential biases and limitations to consider. For example:
- The authors focus primarily on the benefits of using machine learning in asset pricing models but do not discuss any potential drawbacks or risks associated with this approach.
- The article assumes that more accurate asset pricing models are always desirable without considering other factors such as model complexity or interpretability.
- The authors do not provide any evidence to support their claim that machine learning can capture nonlinear relationships between variables better than traditional econometric methods.
- The article does not explore any counterarguments or alternative perspectives on the use of machine learning in finance.
Despite these limitations, the article provides a useful introduction to the topic of machine learning in empirical asset pricing. It highlights some of the key benefits and challenges associated with this approach and provides a starting point for further research.