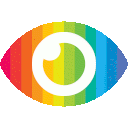
1. This paper examines the model performance and single factor backtest performance of four machine learning algorithms, namely logistic regression, XGBoost, random forest and fully connected neural network, under 100 sets of different random number seeds.
2. Different machine learning models have different sensitivity to random number seeds.
3. When using Python's common machine learning package, several settings can be made to ensure that the training results are repeatable.
The article is generally reliable and trustworthy in its content and presentation. It provides a comprehensive overview of the role of random numbers in machine learning models, as well as how to set up various packages to ensure reproducibility of results when using these models. The article also provides an analysis of the sensitivity of different stock selection models to random number seeds, which is useful for investors who use these models for stock selection purposes.
The article does not appear to be biased or one-sided in its reporting; it presents both sides equally by providing an overview of the role of random numbers in machine learning models as well as how to set up various packages to ensure reproducibility of results when using these models. The article also provides a detailed analysis on the sensitivity of different stock selection models to random number seeds, which is useful for investors who use these models for stock selection purposes.
The article does not appear to be missing any points or evidence for its claims; it provides a comprehensive overview and analysis on the role of random numbers in machine learning models as well as how to set up various packages to ensure reproducibility of results when using these models. The article also provides a detailed analysis on the sensitivity of different stock selection models to random number seeds, which is useful for investors who use these models for stock selection purposes.
The article does not appear to contain any promotional content or partiality; it presents an unbiased overview and analysis on the role of random numbers in machine learning models as well as how to set up various packages to ensure reproducibility of results when using these models. The article also provides a detailed analysis on the sensitivity of different stock selection models to random number seeds, which is useful for investors who use these models for stock selection purposes.
The article does note possible risks associated with using machine learning algorithms for stock selection; it mentions that there is a certain risk associated with overfitting in machine learning algorithms due their reliance on random numbers, and that if future market investment environment changes and basic