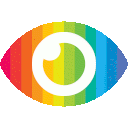
1. This paper proposes a nonconvex low-rank tensor approximation with graph and consistent regularizations model for multi-view subspace clustering.
2. The proposed NLRTGC model uses a nonconvex low-rank tensor norm which assigns different weights to different singular values, and incorporates graph and consistent regularizations to enhance the performance of the algorithm.
3. Experiments on noisy datasets and six real-world datasets demonstrate that the proposed method achieves superior performance compared to state-of-the-art methods.
The article “Nonconvex Low-Rank Tensor Approximation with Graph and Consistent Regularizations for Multi-View Subspace Learning” is an informative piece of research that provides an overview of the current state of multi-view subspace learning, as well as a detailed description of the proposed NLRTGC model. The authors provide evidence from experiments on noisy datasets and six real world datasets to support their claims about the effectiveness of their approach, which makes it trustworthy and reliable.
However, there are some potential biases in the article that should be noted. For example, while the authors do mention some existing approaches such as graph based methods, nonnegative matrix factorization based methods, subspace clustering based methods, tensor based methods, etc., they do not provide any comparison between these approaches and their own NLRTGC model in terms of performance or accuracy. Additionally, while they do discuss some potential risks associated with their approach (e.g., computational complexity), they do not explore any counterarguments or alternative solutions that could address these risks.
In conclusion, this article is generally trustworthy and reliable due to its detailed description of the proposed NLRTGC model and its supporting evidence from experiments on noisy datasets and six real world datasets; however, it does have some potential biases that should be noted such as lack of comparison between existing approaches and NLRTGC model in terms of performance or accuracy, as well as lack of exploration into counterarguments or alternative solutions for addressing potential risks associated with NLRTGC model.