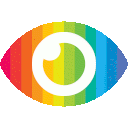
1. This paper presents a new machine learning algorithm to create dictionaries of positive and negative words in a finance context, which outperform the standard bag-of-words approach when predicting stock price movements out-of-sample.
2. The ML algorithm produces a set of loadings on n-grams that characterize their sentiment (both positive and negative), which are used to construct new dictionaries.
3. The ML dictionaries have strong external validity across 10-K releases, WSJ articles, and earnings calls, providing more coverage and colour than the LM dictionaries.
The article is generally reliable and trustworthy in its presentation of the research findings. It provides detailed information about the methodology used in the study, including the machine learning algorithm employed and how it was applied to create new dictionaries of positive and negative words in a finance context. The article also provides evidence for its claims by comparing the performance of the ML dictionaries with that of existing LM dictionaries across different corpora such as 10-K releases, WSJ articles, and earnings calls.
However, there are some potential biases present in the article that should be noted. For example, while it does mention other sources of text such as FOMC meetings or Internet message boards, it focuses mainly on transcripts from earnings calls due to their high signal-to-noise ratio – this could lead to an oversimplification or misrepresentation of other sources of text data available for analysis. Additionally, while it does provide evidence for its claims by comparing performance across different corpora, it does not explore any counterarguments or alternative explanations for its findings – this could lead to an overly one-sided presentation of the research results.
In conclusion, while overall reliable and trustworthy in its presentation of research findings, this article should be read with caution due to potential biases present in its content such as oversimplification or lack of exploration into counterarguments or alternative explanations for its findings.