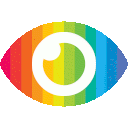
1. The study aimed to find EEG-based features and classification methods that can categorize brainwave patterns based on their level of activity or frequency for mental state recognition useful for human-machine interaction.
2. The Muse headband with four EEG sensors was used to categorize three possible states such as relaxing, neutral, and concentrating based on a few states of mind defined by cognitive behavioral studies.
3. Results show that only 44 features from a set of over 2100 features are necessary when used with classical classifiers such as Bayesian Networks, Support Vector Machines, and Random Forests, attaining an overall accuracy over 87%.
The article titled "A Study on Mental State Classification using EEG-based Brain-Machine Interface" presents a study that aims to find discriminative EEG-based features and appropriate classification methods for mental state recognition useful for human-machine interaction. The study uses the Muse headband with four EEG sensors to categorize three possible states such as relaxing, neutral, and concentrating based on a few states of mind defined by cognitive behavioral studies. The authors have created a dataset with five individuals and sessions lasting one minute for each class of mental state in order to train and test different methods.
The article provides a detailed description of the experimental setup and data acquisition process. However, it lacks information about the potential biases in the study. For instance, the sample size is small, consisting of only five individuals, which may not be representative of the general population. Additionally, there is no mention of any demographic information about the participants, such as age or gender, which could affect their brainwave patterns.
The article claims that only 44 features from a set of over 2100 features are necessary when used with classical classifiers such as Bayesian Networks, Support Vector Machines, and Random Forests, attaining an overall accuracy over 87%. However, there is no evidence provided to support this claim. The authors do not explain how they arrived at this conclusion or provide any statistical analysis to back up their findings.
Furthermore, the article does not explore counterarguments or alternative explanations for their results. For example, it is possible that other factors besides mental state could affect brainwave patterns detected by the Muse headband sensors. Without considering these possibilities or addressing them in their study design or analysis, the authors' conclusions may be limited.
Overall, while the article provides valuable insights into using EEG-based brain-machine interfaces for mental state recognition, it lacks critical analysis and consideration of potential biases and limitations in its methodology and findings.