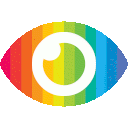
1. The article discusses the potential of using machine learning for designing optimal economic mechanisms, particularly in the field of mechanism design.
2. It highlights the challenges and open questions in mechanism design, such as revenue-optimal auction design for multiple items.
3. The article proposes repurposing machine learning techniques to solve problems of optimal economic design, using training data and loss functions that capture the economic objectives.
The article titled "Machine Learning for Optimal Economic Design" discusses the potential use of machine learning in designing optimal economic mechanisms. While the topic is interesting and relevant, there are several aspects of the article that require critical analysis.
Firstly, the article lacks a clear introduction and background information. It jumps straight into discussing mechanism design without providing sufficient context for readers who may not be familiar with the subject. This makes it difficult to fully understand the significance and implications of using machine learning in this field.
Additionally, the article heavily relies on references to other works and research papers without providing enough explanation or analysis of these sources. This makes it challenging for readers to evaluate the credibility and validity of the claims made in the article. The lack of supporting evidence or empirical data further weakens the arguments presented.
Furthermore, there is a potential bias towards promoting machine learning as a solution to optimal economic design problems. The authors suggest that machine learning can be used to design optimal mechanisms without leveraging characterization results or considering alternative approaches. However, they do not thoroughly explore potential limitations or drawbacks of using machine learning in this context.
The article also fails to address potential risks or ethical considerations associated with automated mechanism design. For example, relying solely on algorithms and computational methods may overlook important social or cultural factors that could impact economic outcomes. Additionally, there may be concerns about transparency and accountability when using machine learning algorithms to make decisions that affect individuals' economic well-being.
Moreover, there is a lack of discussion on potential counterarguments or alternative perspectives. The article presents machine learning as a promising approach but does not adequately consider other existing methods or theories in mechanism design that have been developed over decades of research.
In terms of reporting bias, the article seems to focus primarily on positive aspects and benefits of using machine learning in economic design while downplaying potential challenges or limitations. This one-sided reporting undermines the objectivity and balance of the article.
Overall, while the topic is interesting, the article lacks depth, evidence, and critical analysis. It would benefit from providing a more comprehensive overview of mechanism design, addressing potential limitations and risks of using machine learning, and considering alternative approaches in the field.