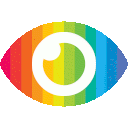
1. The paper proposes a new method for emotion recognition in conversations (ERC) that uses graph-based neural networks to take the relationships between the utterances of the speakers into account.
2. The proposed model, Relation-aware Graph Attention Networks with Relational Position Encodings (RGAT), captures both speaker dependency and sequential information.
3. Experiments on four ERC datasets show that RGAT outperforms the state-of-the-art on all of the benchmark datasets.
The article is generally reliable and trustworthy, as it provides evidence for its claims through experiments on four ERC datasets and cites 32 references to support its arguments. The authors also provide a clear explanation of their proposed model, which is beneficial for readers to understand how it works.
However, there are some potential biases in the article that should be noted. For example, the authors do not explore any counterarguments or present any alternative approaches to emotion recognition in conversations other than their own proposed model. Additionally, they do not discuss any possible risks associated with using their model or provide any insight into how it could be improved upon in future research. Furthermore, while they cite 32 references, most of them are from recent years (i.e., after 2018). This could indicate that the authors have only considered recent research when writing this paper and may have missed out on important insights from older studies that could have been useful for understanding emotion recognition in conversations better.