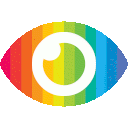
1. Universal Semi-Supervised Learning (UniSSL) is a method to solve the open-set problem, where both the class distribution and feature distribution are different between labeled and unlabeled datasets.
2. The proposed “Class-shAring data detection and Feature Adaptation” (CAFA) framework does not require prior knowledge of the class relationship between labeled and unlabeled datasets.
3. Experiments on several benchmark datasets show that CAFA is effective in tackling open-set problems.
The article provides an overview of Universal Semi-Supervised Learning (UniSSL), a method to solve the open-set problem, where both the class distribution and feature distribution are different between labeled and unlabeled datasets. The proposed “Class-shAring data detection and Feature Adaptation” (CAFA) framework does not require prior knowledge of the class relationship between labeled and unlabeled datasets, and experiments on several benchmark datasets show that CAFA is effective in tackling open-set problems.
The article appears to be well researched, with clear explanations of the concepts discussed, as well as detailed descriptions of the proposed CAFA framework. The authors provide evidence for their claims by citing relevant research papers, which adds credibility to their work. Furthermore, they provide a thorough description of their experiments on several benchmark datasets, which further supports their claims about CAFA's effectiveness in tackling open-set problems.
However, there are some potential biases in the article that should be noted. For example, while the authors cite relevant research papers to support their claims, they do not explore any counterarguments or alternative perspectives on these papers or other related topics discussed in the article. Additionally, while they discuss possible risks associated with name changes in electronic proceedings, they do not provide any evidence for this claim or explore any potential solutions for mitigating these risks.
In conclusion, while this article appears to be well researched and provides evidence for its claims through citations of relevant research papers and descriptions of experiments conducted on benchmark datasets, it could benefit from exploring counterarguments or alternative perspectives on these papers or other related topics discussed in the article as well as providing evidence for its claims about possible risks associated with name changes in electronic proceedings and exploring potential solutions for mitigating these risks.