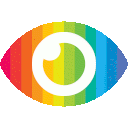
1. The article introduces a novel approach to event schema induction by leveraging large language models (LLMs) to derive event schemas as a form of commonsense knowledge, simplifying the schema induction process.
2. The proposed method utilizes incremental prompting and verification to construct complex event graphs in three stages: event skeleton construction, event expansion, and event-event relation verification, resulting in improved F1 scores for both temporal and hierarchical relations.
3. Compared to previous closed-domain schema induction models, the proposed method outperformed in terms of coverage of events, coherence when translating schemas into stories, and readability ratings by human assessors.
The article titled "Open-Domain Hierarchical Event Schema Induction by Incremental Prompting and Verification" presents a new approach to event schema induction using large language models (LLMs). The authors propose treating event schemas as a form of commonsense knowledge that can be derived from LLMs, simplifying the schema induction process and allowing for handling hierarchical and temporal relations between events.
One potential bias in this article is the focus on the proposed method's strengths without adequately addressing its limitations or potential drawbacks. While the authors highlight improvements in F1 scores for temporal and hierarchical relations compared to previous methods, they do not thoroughly discuss any challenges or shortcomings of their approach. This lack of discussion could lead to an incomplete understanding of the method's effectiveness and applicability in real-world scenarios.
Additionally, the article may exhibit promotional content by emphasizing the superiority of the proposed method over existing approaches without providing a balanced comparison. The authors mention that human assessors rated their schemas higher in terms of readability compared to a previous state-of-the-art model, but they do not provide detailed information on how these assessments were conducted or if there were any biases in the evaluation process.
Furthermore, there is a lack of exploration of potential counterarguments or alternative perspectives on event schema induction. The article focuses solely on the incremental prompting and verification method without discussing other possible approaches or considering different viewpoints within the research community. This narrow focus could limit the reader's understanding of the broader landscape of event schema induction research.
It is also important to note that while the authors claim improvements in F1 scores for temporal and hierarchical relations, there is limited evidence provided to support these claims. The article lacks detailed analysis or validation experiments to demonstrate the robustness and generalizability of the proposed method across different datasets or domains.
Overall, this article may benefit from a more comprehensive discussion of its limitations, potential biases, and alternative perspectives on event schema induction. By addressing these aspects, readers can gain a more nuanced understanding of the proposed method's strengths and weaknesses in relation to existing approaches in the field.