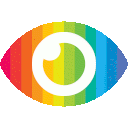
1. Federated Learning (FL) is a technique for training machine learning models on distributed data while maintaining privacy. However, FL consumes a significant amount of communication resources and can compromise participants' private data.
2. The proposed approach, Communication Efficient and Enhanced Privacy (CEEP-FL), aims to minimize communication costs, protect data privacy, and maximize global learning accuracy in FL.
3. The approach includes a filtering mechanism to upload only important gradient updates, Non-Interactive Zero-Knowledge Proofs based Homomorphic-Cryptosystem (NIZKP-HC) for privacy protection, and Distributed Selective Stochastic Gradient Descent (DSSGD) optimization to minimize computational costs. Experimental results show that CEEP-FL outperforms existing approaches in terms of communication efficiency and learning accuracy.
这篇文章介绍了一种名为CEEP-FL的全面方法,旨在提高联邦学习中的通信效率和隐私保护。文章指出,联邦学习在全局模型更新过程中消耗大量的通信资源,并且参与者的私人数据可能会被利用共享参数而泄露,这限制了联邦学习的广泛应用。为了解决这些挑战,作者提出了CEEP-FL方法,该方法同时旨在最小化通信成本、保护数据不被泄露,并最大化全局学习准确性。
文章提到,在减少通信成本方面,他们首先对每个本地梯度更新应用了一种新颖的过滤机制,并只上传重要的梯度。然后,他们采用基于非交互式零知识证明的同态加密系统来保护这些本地梯度更新,同时保持网络的鲁棒性。最后,他们使用分布式选择性随机梯度下降优化来减少计算成本并最大化全局学习准确性。
然而,这篇文章存在一些潜在偏见和不足之处。首先,在介绍现有方法时,并没有充分讨论它们的优点和贡献。其次,文章没有提供足够的证据来支持他们所提出的方法在通信成本和隐私保护方面的优势。虽然作者进行了实验来评估他们的方法,但并没有详细说明实验设置和结果。此外,文章没有探讨可能存在的风险和潜在问题,并且没有平等地呈现双方观点。
总体而言,这篇文章提出了一个有潜力的方法来改善联邦学习中的通信效率和隐私保护,但需要更多的实验证据和全面考虑可能存在的问题和风险。