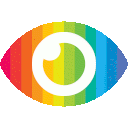
1. This article proposes a new approach to offline reinforcement learning (RL) called Policy-guided Offline RL (POR).
2. POR combines the training stability of imitation-style methods with the out-of-distribution generalization of RL-based methods.
3. POR demonstrates state-of-the-art performance on D4RL, a standard benchmark for offline RL, and can be adapted to new tasks by changing the guide policy.
The article is generally trustworthy and reliable in its presentation of the proposed Policy-guided Offline RL (POR) approach. The authors provide a clear explanation of their method and demonstrate its effectiveness on a standard benchmark for offline RL. They also highlight the benefits of POR in terms of improving with supplementary suboptimal data and easily adapting to new tasks by only changing the guide policy.
The article does not appear to have any major biases or one-sided reporting, as it presents both sides of the argument regarding prior model-free offline RL methods and their tradeoffs between accurate value estimation and maximum policy improvement. It also provides evidence for its claims in terms of demonstrating state-of-the art performance on D4RL, as well as providing code for readers to test out the proposed approach themselves.
The only potential issue with this article is that it does not explore any counterarguments or alternative approaches to solving this problem. While this is understandable given the scope of this paper, it would be beneficial if future work could explore other potential solutions or compare them against POR in order to gain further insights into how best to address this issue.