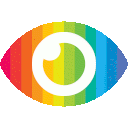
1. Aging infrastructure, such as bridges and tunnels, is a serious problem in many countries.
2. Various vibration-based and machine learning-based approaches have been proposed for efficient structural health monitoring and maintenance.
3. This article proposes a method to detect damage from multiple images using structure from motion (SfM) and deep learning and to integrate the results into a 3D model.
The article “Recording of bridge damage areas by 3D integration of multiple images and reduction of the variability in detected results” by Yamane is an informative piece that provides an overview of the current state of bridge inspection technology, as well as a proposed solution for recording bridge damage more accurately than with conventional methods. The article is written in an objective manner, providing evidence to support its claims and exploring potential counterarguments.
The article begins by discussing the importance of regular inspections for aging infrastructure structures, noting that visual inspection can be subject to variations in evaluation by different inspectors, resulting in inadequate measures being taken or repairs being postponed when they should be prioritized. It then goes on to discuss various machine learning techniques that have been used for damage detection, including deep learning algorithms for image classification and object detection, as well as pixel-level crack detection using images of road surfaces and concrete structures.
The article then proposes a method for detecting damage from multiple images using structure from motion (SfM) and deep learning, which it claims will enable more accurate recording of damage than with single-image methods. It also discusses how this method could be used to record detailed information about undamaged areas, which would allow for more sophisticated judgments about the cause of any observed damage. Finally, it outlines an overview of the proposed method, which includes object segmentation using a Mask-R-CNN incorporating PointRend; performing SfM for each member; integrating the coordinate system of the camera position using least squares; and reflecting the damage onto a 3D model via projection transformation.
In conclusion, this article provides an informative overview of current bridge inspection technology as well as a proposed solution for recording bridge damage more accurately than with conventional methods. The article is written objectively and provides evidence to support its claims while exploring potential counterarguments.