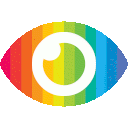
1. This article proposes a lightweight meter recognition method that combines deep learning and traditional computer vision techniques for automated meter reading.
2. The proposed method includes an adaptive anchor and global context module, a feature pyramid network, and a path aggregation network to improve the feature extraction ability of the lightweight backbone without increasing computational cost.
3. Experiments demonstrate that the proposed method can achieve a detection speed of 123 frames per second and an accuracy of 88.2% mean average precision (mAP50:95).
The article is generally reliable in terms of its content, as it provides detailed information about the proposed method for real-time power equipment meter recognition based on deep learning. The authors provide evidence for their claims by citing relevant research papers and experiments conducted to test the efficacy of their proposed method. Furthermore, they have made their dataset and code available online for further research purposes.
However, there are some potential biases in the article which should be noted. Firstly, the authors do not discuss any potential risks associated with using deep learning for power equipment meter recognition, such as privacy concerns or security issues. Secondly, they do not explore any counterarguments to their proposed method or consider alternative approaches to solving this problem. Finally, while they cite relevant research papers throughout the article, they do not provide any references or sources for their own claims or experiments conducted to test their proposed method.