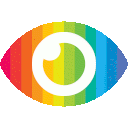
1. Stock market prediction is a challenging task due to the non-stationary, nonlinear, and volatile nature of financial time series data.
2. Deep learning algorithms, such as LSTM and CNN, have been applied in stock market analysis with good results.
3. Frequency decomposition methods, such as EMD and CEEMD, can be combined with deep learning models to improve their performance in analyzing decomposed time-series data. The proposed CEEMD-CNN-LSTM algorithm shows promise in predicting stock prices accurately.
The article titled "Stock price prediction using deep learning and frequency decomposition" discusses the use of deep learning models, specifically LSTM and CNN, in predicting stock market trends. The article also explores the use of frequency decomposition algorithms such as EMD and CEEMD to reduce the complexity of data series.
The article provides a comprehensive review of related literature on the application of deep learning models in stock market analysis. It also highlights the challenges associated with financial time series data, including non-stationarity, nonlinearity, and high volatility. The authors argue that while precise forecasting of markets is impossible, recent advances in computing technology have made it possible to predict stock market trends with reasonable accuracy.
The methodology section explains the constituting models used in this study, including CNN, LSTM, CNN-LSTM, EMD, and CEEMD. The authors propose a hybrid algorithm called CEEMD-CNN-LSTM for analyzing stock market data. They evaluate this algorithm using data from various international stock price indices and compare its performance with other models.
While the article provides valuable insights into the use of deep learning models and frequency decomposition algorithms in predicting stock market trends, there are some potential biases and limitations to consider. For example, the authors do not discuss the limitations of their proposed algorithm or acknowledge any potential risks associated with relying on machine learning models for financial decision-making.
Additionally, while the article provides a comprehensive review of related literature on deep learning models in finance, it does not explore counterarguments or alternative perspectives on this topic. This one-sided reporting may limit readers' understanding of the broader debate surrounding machine learning's role in finance.
Overall, while this article provides valuable insights into using deep learning models and frequency decomposition algorithms for predicting stock market trends, readers should approach its claims with caution and consider alternative perspectives before making any financial decisions based on these findings.