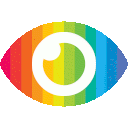
1. A deep learning method based on a deep auto-encoder (DAE) network is proposed for anomaly detection and fault analysis of wind turbine components.
2. An adaptive threshold determined by the extreme value theory is used as the rule of anomaly judgement to avoid false alarms caused by abrupt changes in wind speed.
3. The effectiveness of the proposed method was verified by some reported failure cases of wind turbine components.
The article “Anomaly Detection and Fault Analysis of Wind Turbine Components Based on Deep Learning Network” provides an overview of a deep learning method for detecting anomalies and faults in wind turbines. The article is well written and provides a clear explanation of the proposed method, which includes using a deep auto-encoder (DAE) network to construct a condition index Re, an adaptive threshold based on extreme value theory, and residuals from the DAE network model for fault analysis and location. The article also presents case studies to demonstrate the effectiveness of the proposed method.
However, there are some potential biases that should be noted when evaluating this article. First, it does not provide any evidence or data to support its claims about the effectiveness of the proposed method; instead, it relies solely on case studies which may not be representative enough to draw conclusions about its efficacy. Additionally, there is no discussion about possible risks associated with using this method or any counterarguments that could be made against it. Furthermore, while the article does mention some limitations such as false alarms caused by abrupt changes in wind speed, it does not explore other potential issues that could arise from using this approach such as overfitting or incorrect assumptions about data distributions. Finally, there is no discussion about alternative methods that could be used for anomaly detection and fault analysis in wind turbines which could provide additional insights into this topic.
In conclusion, while this article provides an interesting overview of a deep learning approach for detecting anomalies and faults in wind turbines, it should be evaluated critically due to potential biases such as lack of evidence for its claims and missing points of consideration regarding possible risks or alternative methods that could be used instead.