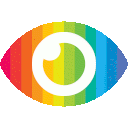
1. Large artificial neural networks trained via self-supervision can learn major aspects of linguistic structure, including syntactic grammatical relationships and anaphoric coreference, without explicit supervision.
2. These models, such as BERT, develop rich hierarchical structures by predicting masked words in a given context, leading to improved language understanding across various tasks.
3. The success of these models in learning language structure from positive evidence alone challenges the traditional approach of hand-labeling linguistic representations and suggests that large-scale syntactically labeled training data may no longer be necessary for many tasks in natural language processing.
The article "Emergent linguistic structure in artificial neural networks trained by self-supervision" published in PNAS explores the knowledge of linguistic structure learned by large artificial neural networks trained via self-supervision. The paper discusses how modern deep contextual language models, such as BERT, are able to learn major aspects of linguistic structure without explicit supervision. The authors demonstrate that these models capture syntactic grammatical relationships and anaphoric coreference, showing a surprising ability to approximate sentence tree structures proposed by linguists.
One potential bias in the article is the focus on the positive aspects of self-supervised learning and the capabilities of artificial neural networks in capturing linguistic structure. While the results presented are indeed intriguing and significant, there may be limitations or drawbacks to this approach that are not fully explored or acknowledged. For example, the article does not discuss potential challenges or failures of self-supervised learning in capturing complex linguistic phenomena accurately.
Additionally, the article may present a somewhat one-sided view of the effectiveness of large-scale artificial neural network models in language understanding. While the authors highlight the success of these models in capturing syntactic and semantic information, they do not delve into potential criticisms or limitations of relying solely on machine learning approaches for language understanding. It would be beneficial to include a discussion on the interpretability of these models, potential biases encoded in their training data, and ethical considerations related to their deployment.
Furthermore, while the article provides detailed explanations of how BERT and other Transformer models work, it lacks concrete evidence or empirical studies to support some of its claims about the capabilities of these models. For instance, while it is stated that BERT can capture parse tree distances to a surprising degree, more evidence or experiments demonstrating this would strengthen the argument.
The article also appears to have a promotional tone towards self-supervised learning and Transformer models like BERT. While it is important to highlight advancements in natural language processing research, it is essential to maintain objectivity and provide a balanced perspective on both the strengths and limitations of these technologies.
In conclusion, while "Emergent linguistic structure in artificial neural networks trained by self-supervision" presents valuable insights into how modern deep contextual language models learn linguistic structure without explicit supervision, there are areas where further exploration and critical analysis could enhance the depth and credibility of the findings presented. By addressing potential biases, acknowledging limitations, providing supporting evidence for claims made, and presenting a more balanced view of machine learning approaches in language understanding, future research can build upon this work effectively.