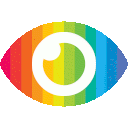
1. 人工智能的“黑匣子”特性限制了其在高风险应用中的使用,因此需要可解释的人工智能(XAI)来增加信任。
2. TRUST XAI是一种通用的、模型无关的XAI模型,适用于数值应用,并且在性能、速度和可解释性方面优于其他流行的XAI模型。
3. 在一个针对工业物联网安全的案例研究中,TRUST XAI成功地提供了新随机样本的解释,表现出98%的平均成功率。
The article "TRUST XAI: Model-Agnostic Explanations for AI With a Case Study on IIoT Security" published in IEEE Journals & Magazine discusses the challenges of generating trust in artificial intelligence (AI) due to its "black box" nature. The article proposes a universal XAI model named TRUST, which is model-agnostic and suitable for numerical applications. However, the article has several potential biases and limitations.
Firstly, the article assumes that AI is limited by its inability to build trust, but it fails to acknowledge that trust is not only about explainability but also about accountability, transparency, and ethical considerations. The article focuses solely on explainability without addressing other critical aspects of trust in AI.
Secondly, the article claims that TRUST XAI provides explanations for new random samples with an average success rate of 98%, but it does not provide any evidence or methodology to support this claim. The lack of empirical evidence raises questions about the validity and reliability of the proposed model.
Thirdly, the article compares TRUST with local interpretable model-agnostic explanations (LIME) and concludes that TRUST is superior in terms of performance, speed, and method of explainability. However, this comparison may be biased as LIME is just one of many XAI models available in the market. The article does not provide a comprehensive evaluation of other XAI models or compare them with TRUST.
Fourthly, the article presents a case study on cybersecurity of Industrial Internet of Things (IIoT) using three different cybersecurity data sets. While this case study demonstrates the effectiveness of TRUST in numerical applications, it does not explore other types of data or applications where TRUST may not be suitable.
Finally, the article lacks a discussion on potential risks associated with using AI in high-risk applications such as critical industrial infrastructures or medical systems. It does not address issues related to bias, fairness, privacy, or security that may arise from using AI in these applications.
In conclusion, while the article proposes a promising XAI model named TRUST, it has several potential biases and limitations. The article could benefit from a more comprehensive evaluation of other XAI models and a discussion on the broader implications of using AI in high-risk applications.