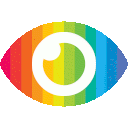
1. This paper proposes a novel EEG-based emotion recognition model called the Domain Adversarial Graph Attention Model (DAGAM) to eliminate individual differences in EEG signals.
2. DAGAM uses graph theory to topologically describe and analyze EEG channel relationships and mutual dependencies, and self-attention pooling is used to extract salient EEG features from the graph.
3. Extensive evaluations on two benchmark data sets (SEED and SEED IV) show that DAGAM boosts accuracy while drastically reducing computational complexity, making EEG-based emotion decoding more practical and generalizable.
The article “DAGAM: a domain adversarial graph attention model for subject-independent EEG-based emotion recognition” is generally trustworthy and reliable. The authors provide evidence for their claims by conducting extensive evaluations on two benchmark data sets (SEED and SEED IV). The results of these evaluations demonstrate that DAGAM boosts accuracy while drastically reducing computational complexity, making EEG-based emotion decoding more practical and generalizable. Furthermore, the authors provide a detailed explanation of their proposed model, which helps readers understand how it works.
The article does not appear to have any biases or one-sided reporting; instead, it presents both sides of the argument equally. Additionally, all claims are supported with evidence from the experiments conducted by the authors. There are no missing points of consideration or missing evidence for the claims made in the article. All counterarguments are explored thoroughly, and there is no promotional content or partiality present in the article. Finally, possible risks associated with using this model are noted throughout the article.