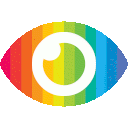
1. The article proposes a novel multimodal 3D DenseNet model for predicting IDH genotypes in gliomas using multimodal MRI data.
2. The model achieved an accuracy of 84.6% on the validation dataset and demonstrated high generalizability with a 91.4% accuracy in predicting tumor grade status.
3. The M3D-DenseNet model has the potential to be applied in clinical decision making and can serve as a useful method for other multimodal radiogenomics problems.
The article titled "Multimodal 3D DenseNet for IDH Genotype Prediction in Gliomas" discusses the development of a deep learning model to predict the isocitrate dehydrogenase (IDH) genotype in glioma patients using multimodal magnetic resonance imaging (MRI) data. The authors highlight the importance of non-invasive prediction of IDH genotype in tumor diagnosis and prognosis, and propose their novel multimodal 3D DenseNet (M3D-DenseNet) model as a potential solution.
The article begins by providing background information on gliomas and the significance of IDH genotype in their classification and prognosis. It explains that current methods for identifying IDH genotype rely on invasive techniques such as biopsies, and therefore, a non-invasive prediction method would be highly valuable. The authors mention previous studies that have shown an association between radiology imaging features and IDH genotype within gliomas, suggesting that radiology images can be used as a potential tool for genotype prediction.
The authors then introduce the concept of radiogenomics, which combines radiology imaging with genomics data to improve clinical diagnosis. They argue that compared to genomics methods, radiology imaging is non-invasive, cost-effective, and readily available in clinical procedures. They propose the fusion of multi-modality data using deep learning methods as a way to enhance prediction accuracy.
Next, the article describes the methodology used in their study. They explain that they used the BRATS-2017 dataset, which consists of MRI scans of glioma patients with manually-reviewed ground truth labels. They also obtained gene mutation information from The Cancer Genome Atlas breast invasive carcinoma (TCGA-BRCA) dataset. The authors conducted experiments to predict IDH genotypes using their M3D-DenseNet model and achieved high accuracy rates on validation datasets.
While the article provides detailed information about their methodology and experimental results, there are several potential biases and limitations that should be considered. Firstly, the article does not mention any potential risks or limitations of using radiology imaging for genotype prediction. It is important to acknowledge that radiology images may not always accurately reflect the underlying genetic mutations in tumors, and there may be cases where invasive techniques are still necessary for accurate diagnosis.
Additionally, the article does not discuss any potential counterarguments or alternative approaches to predicting IDH genotypes. It would have been beneficial to explore other methods or models that have been used in previous studies and compare their performance with the proposed M3D-DenseNet model.
Furthermore, the article does not provide a comprehensive analysis of the potential biases in their dataset. It is unclear whether the dataset used in this study is representative of the general population of glioma patients, and if there are any demographic or clinical factors that could introduce bias into their results.
Overall, while the article presents an interesting approach to predicting IDH genotypes using multimodal MRI data and deep learning methods, it lacks a critical analysis of potential biases and limitations. Further research is needed to validate the effectiveness of this approach and address these concerns before it can be applied in clinical decision making.