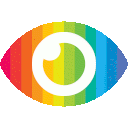
1. Adequate statistical power is essential for observing true relationships in a dataset, and a thoughtful power analysis helps determine the appropriate sample size for an analysis in EFA, CFA, and SEM studies.
2. Factors such as Alpha, Beta, statistical power, and Effect size play a crucial role in determining the sample size needed for a study, with larger samples generally leading to more stable factor structures in EFA.
3. While it is important to have an adequate sample size for accurate parameter estimates in SEM and factor analysis, having an excessively large sample can lead to overstating unimportant effects and wasting research effort.
The article provides a comprehensive overview of the importance of sample size and sample power considerations in factor analysis (EFA, CFA) and SEM studies. It discusses the significance of statistical power in detecting true relationships in a dataset and emphasizes the need for adequate but not excessive sample sizes. The article also delves into the parameters related to power analysis, such as Alpha, Beta, statistical power, and Effect size, which are essential for determining the appropriate sample size.
One potential bias in the article is its focus on the importance of statistical power without adequately addressing potential risks associated with underpowered studies. While it mentions that inadequate sample sizes can lead to failure to detect significant effects, it does not delve into the consequences of Type II errors (false negatives) or the implications of drawing incorrect conclusions due to low statistical power.
Additionally, the article primarily discusses the benefits of having an optimal sample size for accurate analysis but does not thoroughly explore the challenges or limitations associated with obtaining large samples. It briefly mentions that unduly large samples could overstate unimportant effects but does not provide a detailed discussion on how researchers can strike a balance between sample size and research costs.
Furthermore, while the article touches upon different methodologies for estimating statistical power and sample size before data collection (a priori analysis), it does not delve into post-hoc or retrospective power analysis. Exploring both pre- and post-data collection power analyses could provide a more comprehensive understanding of how researchers can assess and adjust their study designs based on actual data outcomes.
The article also lacks discussion on potential biases or confounding factors that may influence sample size considerations in factor analysis and SEM studies. Factors such as sampling methods, participant demographics, measurement error, and model complexity could impact the adequacy of sample sizes but are not extensively addressed in the article.
Overall, while the article provides valuable insights into sample size and power considerations in psychometric studies, it could benefit from a more balanced discussion on the risks and limitations associated with different sample sizes, as well as a deeper exploration of methodological considerations for estimating statistical power. Additionally, addressing potential biases or confounding factors that may affect sample size decisions would enhance the overall credibility and applicability of the information presented.