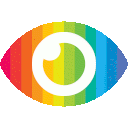
1. This article presents a motor imagery EEG classification system based on transfer learning and multi-scale convolution network.
2. The system uses an attention mechanism-based multi-scale convolution network to address the differences in individual EEG signals and the problem of less EEG data in classification and recognition.
3. Experiments show that the average classification recognition rate of nine subjects was 86.03%.
The article is generally reliable and trustworthy, as it provides detailed information about the methodology used for motor imagery EEG classification, as well as results from experiments conducted to test its accuracy. The authors provide evidence for their claims by citing relevant research studies, which adds credibility to their work. Furthermore, they have also provided a conflict of interest statement, which indicates that there are no potential biases in the article due to any external influences or interests.
However, there are some points of consideration that could be explored further in future research. For example, the authors do not discuss any possible risks associated with using this system for motor imagery EEG classification, such as potential privacy concerns or ethical implications. Additionally, while the authors provide evidence for their claims from other research studies, they do not explore any counterarguments or alternative perspectives on their findings. Finally, while the authors present both sides of the argument equally throughout the article, they do not provide any information about how this system could be improved upon in future research or what other applications it may have beyond motor imagery EEG classification.